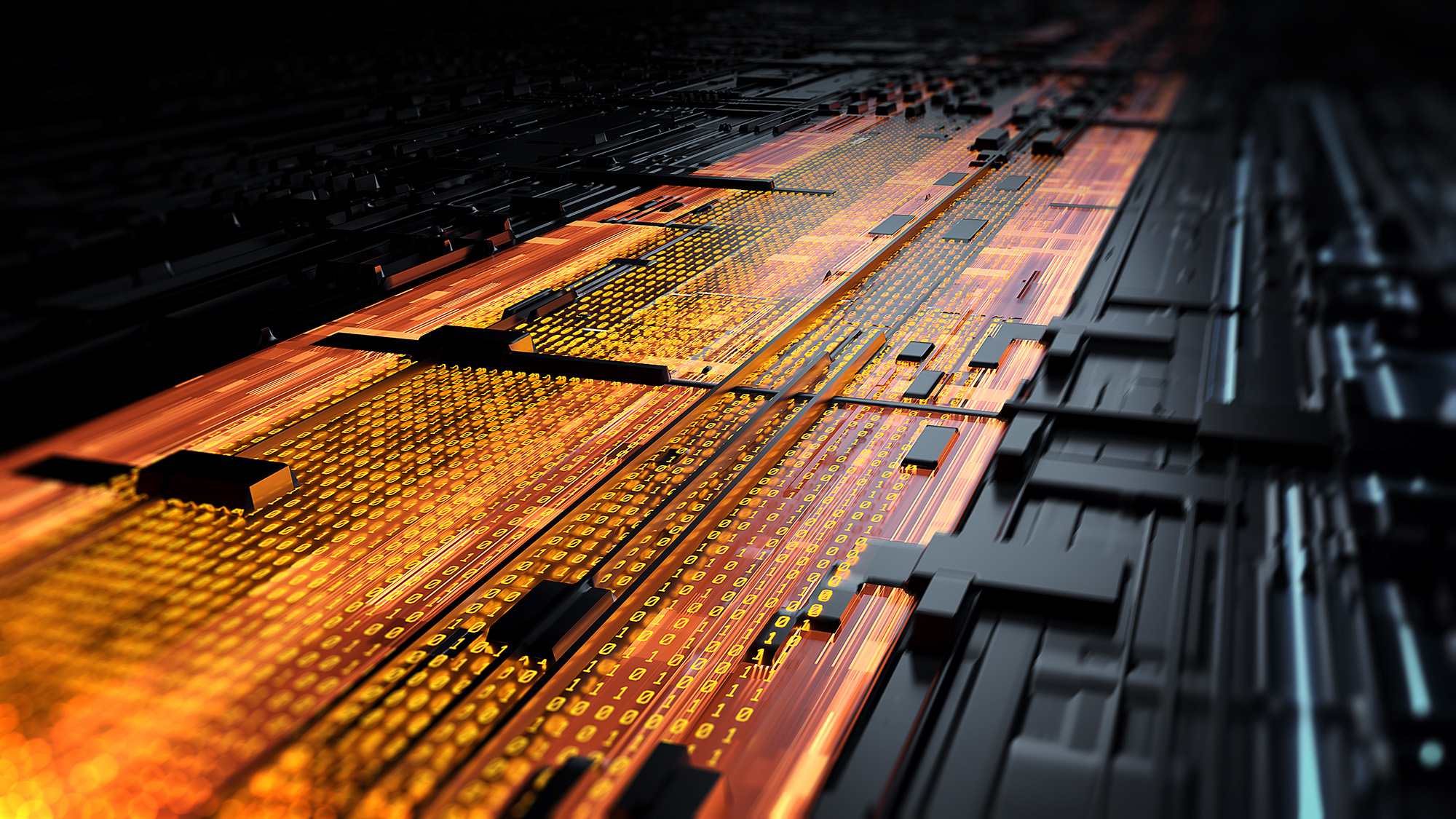
IPCEI
What our mind focuses on
We are helping our customers utilizing the data they already collect to keep their grids stable in the era of decentralization and decarbonization.
Visual Grid Analytics
Smart grids produce large amounts of data that can be used in many different ways. But even having the access to all the analytical insights, charts and spreadsheets, it’s easy to get lost or fail to see the big picture. That’s why Mycroft Mind came up with a new way of translating such complex inputs into a comprehensible output – the smart grid digital twin.
Five-star data processing
maturity rating
Data Collection
Smart meters and sensors provide a continuous stream of millions of measurements and events. The sole function of a one star system is to cleanse, validate and store. A one star system works very much like a data warehouse, allowing data to be stored and queried. This is a necessary function although insufficient to allow the most effective use to be made of any data measured. Querying data, which grows daily by hundreds of millions of measurements, is no easy task for users either.
Filtering
Filtering is the simplest way of processing data. It creates an event when the measurement (e.g. voltage) is either higher or lower than the threshold defined; A two star system can both filter and alert. Would users, however, want to be confronted with thousands of individual alerts each hour?
Detection
A system deserves a three star rating when it is able to detect clusters of related events, analyze data in relation to grid topology and detect situation and behaviour patterns. For example to inform users about a specific substation feeder which has been experiencing repeated voltage problems since last Spring which occurs when a specific photovoltaic source is generating a lot of power.
Prediction
A four star data processing system will warn you when an incident is about to happen. Being able to predict consumption, production and reaction to changing levels of demand is vital for efficient and effective grid management. Accurate information of this kind is key to switch from a reactive to a proactive mode of control.
Adaption
Grid behaviour is continuously evolving and changing. New behaviour patterns occur, priorities evolve and new threads arise. Fixed data processing rules are very soon out of date. Adaptive algorithms are necessary to detect and understand all changes and anomalies.
150 000 measurement/s
prediction speed
3.5mil devices
in simulation environment
1M rows 30 columns/12s
machine learning speed
11 billions
smart meter measurements analyzed
60 000/s
measurements processed
15%
better estimation algorithm than standardly used